
Good Data Management Practices is the Key to Successful AI

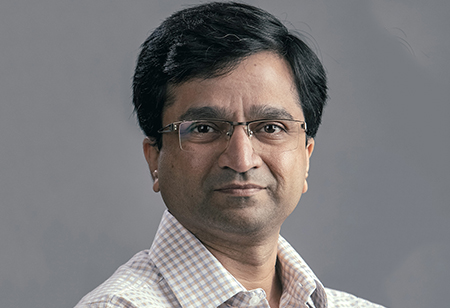
Milind Borate, Co-Founder & CTO, Druva
To realize the dream of AI of things, algorithms must be trained on the correct set of data and simple decision-making models must be created.
Data Management & AI
The relation between AI and data is very symbiotic. Good machine learning needs good data, and with ever-evolving data, machine learning is becoming the key to understand data better. Machine learning and data can be used to crack the code of success beyond rough modeling, ensure a company is properly prepared for future growth, or even see around a corner to navigate upcoming challenges.
The ability to leverage nearly limitless compute and storage capability in the cloud provide businesses a way to leverage more data for business needs. Using AI capabilities, data can offer insights into sales projections, customer demands, and even internal HR needs. Meanwhile, regulations like GDPR, retention policies, cleaning & normalizing data, and data problems are only magnified as data foot-prints increase. This further magnifies the potential of AI to stream-line rote business processes. There are thousands of tasks that need to be done consistently to properly manage data so that it can actually be put to use.
At the same time, businesses face the critical challenge of increasing their awareness of the data environment. Architecting data centers is a dying art, and there is little incentive for an enterprise to retain skills when infrastructure is refreshed once a decade. People who built the applications and the data architecture are ever rarer, and the next generation of talent is not focused on those skillsets.
Additionally, as businesses increasingly adopt cloud services, data silos are only growing. Getting data to a single repository, like a data lake, has be-come a chief challenge and is essential to applying AI on a broader scale. Today, data collection through backup casts an overly wide net based on complex policies determining what is captured. Ask an IT per-son how much data they own or even care about, and they probably cannot give a reliable answer. There are just too many parties, privacy concerns, storage considerations, permissions, and collection requirements for most companies to have a definitive grasp on their data.
So how do we prepare for this new reality, where data is more important than ever and yet the number of data experts on staff is quickly shrinking? Fortunately, the need for excellent data management and the emergence of AI are complementary.
Leveraging AI for Data Needs
One solution is to leverage the emerging AI of Things the application of commoditized, cloud-based Machine Learning to consistently and continually automate millions of small decisions about data. Just as the Internet of Things pulls in data from the smallest sensors, so the AI of Things uses AI and Machine Learning to execute myriad repetitive tasks such as ensuring that data retention policies are appropriately enforced. This is exactly the sort of thing that AI can help us with: providing consistency in the handling of millions of small, but crucial data management tasks. Empowering your teams with such AI opens-up a world of possibilities. Your IT team is able to automate tasks, while your business teams have access to more self-service features. All of this helps streamline the process, further leverages your most insightful data, and empowers your teams to make decisions based on sound data.
By applying AI to the various, myriad minute processes related to handling data, we can increase the overall intelligence of the software storing this data, managing the data lake, and updating as necessary. Just like our consumer devices, we can distribute small-scale AI functionality to handle very granular objects, automating backup, controlling access, granting & revoking permissions, enforcing security, and more, which in the aggregate, make the entire system smarter and less unwieldy. Best of all, this is far simpler than the traditional rebuild and re-architect approach.
Adopting an evolutionary approach that improves lower-level services firstly is the key to enhancing higher level, AI-driven decision-making and unlocking the full potential of organizational data. Before you build your house, you need to ensure you have a solid foundation, and focusing AI on these processes does just that. Then, enterprises can turn their attention from using AI to solve base-level problems, to using this powerful technology to position a company for long-term, enterprise-scale AI success.
So how do we prepare for this new reality, where data is more important than ever and yet the number of data experts on staff is quickly shrinking? Fortunately, the need for excellent data management and the emergence of AI are complementary.
Leveraging AI for Data Needs
One solution is to leverage the emerging AI of Things the application of commoditized, cloud-based Machine Learning to consistently and continually automate millions of small decisions about data. Just as the Internet of Things pulls in data from the smallest sensors, so the AI of Things uses AI and Machine Learning to execute myriad repetitive tasks such as ensuring that data retention policies are appropriately enforced. This is exactly the sort of thing that AI can help us with: providing consistency in the handling of millions of small, but crucial data management tasks. Empowering your teams with such AI opens-up a world of possibilities. Your IT team is able to automate tasks, while your business teams have access to more self-service features. All of this helps streamline the process, further leverages your most insightful data, and empowers your teams to make decisions based on sound data.
By applying AI to the various, myriad minute processes related to handling data, we can increase the overall intelligence of the software storing this data, managing the data lake, and updating as necessary. Just like our consumer devices, we can distribute small-scale AI functionality to handle very granular objects, automating backup, controlling access, granting & revoking permissions, enforcing security, and more, which in the aggregate, make the entire system smarter and less unwieldy. Best of all, this is far simpler than the traditional rebuild and re-architect approach.
Adopting an evolutionary approach that improves lower-level services firstly is the key to enhancing higher level, AI-driven decision-making and unlocking the full potential of organizational data. Before you build your house, you need to ensure you have a solid foundation, and focusing AI on these processes does just that. Then, enterprises can turn their attention from using AI to solve base-level problems, to using this powerful technology to position a company for long-term, enterprise-scale AI success.