
Current Trends in Big Data & Data Mining, with Applications to Robotics

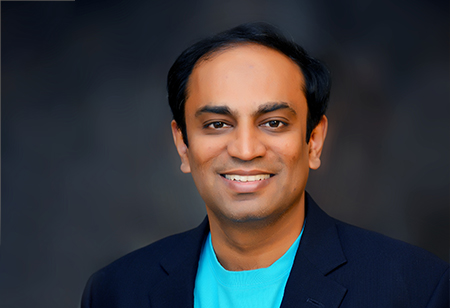
Ramakrishnan Ramanathaiah, Director- SAP Practices, Miraclesoft
Data mining has found applications in a wide range of industrial sectors and one of cutting edge sectors which is being changed by data science is the field of autonomy. A device is referred to as being autonomous if that device carries out tasks with no or very limited human intervention. Autonomy is a cutting edge area of research that promises to unlock enormous productivity gains and enable humans to focus more on tasks that they are best suited for. This will increase the average standard of living for the vast majority of the population and also increase wealth. Areas where autonomy is currently being intensively explored includes application areas such as transport (autonomous vehicles) and manufacturing (autonomous robots). In transport, the goal is to have vehicles drive themselves without human intervention and in manufacturing the goal is to have robotic arms take on a lot more tasks in the manufacturing sector which are currently being done by human operators.
The role that big data plays in the development of these areas is enormous. In autonomy for instance, the manner in which an autonomous system is developed is very incremental and data driven. So a prototype of an autonomous robot would be first developed and then tested in a real world scenario, the various outputs from the numerous sensors that are on the robot would then produce a lot of data and that data needs to used to inform design decisions. As the number of sensors increases, it becomes increasing difficult for conventional data processing techniques to handle the data techniques and simpler more generic data mining techniques need to be used instead.
Using data from the sensors, we can iteratively improve the performance of the system by using data mining tools to find patterns from the mass amounts of data that a device produces and make gain information from it. This allows the development of cutting edge technological advances that leverage big data to improve their performance in time.
A concrete example of the advances that have come about in robotics as a consequence of data mining
can be seen in cutting edge development of automated industrial robotic arms that self diagnose faults. Typically, in a industrial setting robotic arms are used on the production line to improve efficiency. These robotic arms do a wide variety of tasks such as fitting various fixtures and fitting to machines. Due to the complexity of these machines, when they malfunction in some way it may be difficult to notice that they are malfunctioning until they have done a lot of damage (a malfunction could be something as small as losing precision). Once a robotic arm has been identified as malfunctioning in some way, it may take a long time to find the reason of the malfunction as scan tools might only throw up generic error codes.
Data mining allows us to focus on just looking for patterns in data and comparing those patterns to other patterns we have seen in the past. It is because of this system wide view of system produced data that has enabled the field to be so successful. Instead of concentrating on understanding and having a clear idea of every single data sample that we have access to, we just focus on the major trends and patterns in the data, these patterns turn out to be the most important elements in the data in most instances.
The example highlighted in this article of the use of big data in state of the art robotic systems is an indication of the kind of impact advances in this field are having in the manufacturing and industrial economy. With the use of digital devices continuing to increase year upon year and the increasing connectedness of devices (such as is the case with IoT), data will continue to be generated at increasing rates and data mining techniques will be necessary to make sense of all the data that is being produced.
Data mining is being used in cutting edge systems to help mitigate the issue that is high- lighted above. Data produced by the wide variety of sensors that are present on the robotic arm is constantly being analysed in real-time with the aim of detecting anomalies. Once an anomaly is detected, the issue is investigated further by proceeding to ascertain the source of the anomaly. This could be via comparative approaches where the abnormal data is analysed and compared with data produced by other robotic arms to find similar. Techniques such as these and also statistical techniques are used to automatically diagnose and suggest possible remedies. After this, a remedy is suggested, it is up to the engineer in charge of the robotic arm to assess the suggested remedy and chose whether to implement. This whole process helps malfunctioning robotic arms to be identified and fixed as quickly as possible. This then helps to improve productivity and hence profitability.Autonomy is a cutting edge area of research that promises to unlock enormous productivity gains and enable humans to focus more on tasks that they are best suited for
Data mining allows us to focus on just looking for patterns in data and comparing those patterns to other patterns we have seen in the past. It is because of this system wide view of system produced data that has enabled the field to be so successful. Instead of concentrating on understanding and having a clear idea of every single data sample that we have access to, we just focus on the major trends and patterns in the data, these patterns turn out to be the most important elements in the data in most instances.
The example highlighted in this article of the use of big data in state of the art robotic systems is an indication of the kind of impact advances in this field are having in the manufacturing and industrial economy. With the use of digital devices continuing to increase year upon year and the increasing connectedness of devices (such as is the case with IoT), data will continue to be generated at increasing rates and data mining techniques will be necessary to make sense of all the data that is being produced.