
The Role of Data Science and Predictive Analytics in Banking

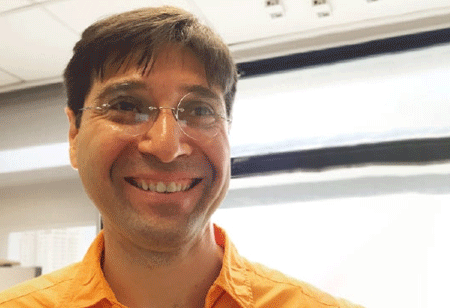
Marcelo Labre, Head of Analytics and Market Data, OCBC Bank
In my 15 years of working with quantitative and data analytics in banks, I have watched some wasteful actions such as IT departments purging data simply because of the need for storage space, despite the increasingly low cost of storage hardware. Yet, I have also witnessed a gradual increase of interest in banks to tap their vast idle data resource. This is evident in the number of top tier banks adding Chief Data or Chief Analytics Officer roles to their C-suite, as well as the multiplication of industry events focusing on data and geared toward financial institutions.
The fact of the matter is that banks are still way behind the curve when it comes to effective use of data. One reason for this could be because these are highly regulated entities where innovation is much harder to achieve than in the less regulated sectors. Given how critical some of banks’ internal technology systems are, there is also a natural lag in new IT infrastructure development. And yet another contributor is that the areas mostly in need of innovation through data analytics are still dominated by the dogma of mathematical finance, which dismisses any solutions not resembling a “Brownian Motion” approach, even if the new approach fits reality more accurately.
Regardless of the obstacles, the way forward is as simple as getting it started. I was fortunate to lead a few novel initiatives applying machine learning and predictive analytics to areas previously not considered as data science applications. One instance relates to assessing default risk of non-rated bonds in a bank’s portfolio, a problem all banks have to a larger or lesser extent. Bonds are like loans, where an entity issues paper in exchange for borrowing a principal amount with the promise to pay back at a certain expiration date, plus regular interest installments. As market participants regularly trade these financial instruments, an assessment of default risk is paramount for valuations and risk management. But in the case of banks, the story is more complicated.
Regulators require that financial institutions set aside a certain reserve of cash in the form of regulatory capital. Given that banks make a living off deploying capital (into loans, securities underwriting, etc), this reserve is supposed to create an overall safer financial system by restricting the bets these institutions can make with that capital. On the other hand, the more capital a bank has available for business rather than stuck as a regulatory reserve, the more profitable it becomes. Striking a balance between risk taking and safety is one of the major challenges for financial institutions. This played out vividly during the global financial crisis of 2007-2009.
Regulatory capital on risk taking from bonds is calculated in line with the risk of the debtor to default on its obligations. This risk is normally assessed via the credit ratings assigned to those bonds by one of the three major rating agencies, Standard & Poors’, Moody’s and Fitch, comes in the form of categories such as AAA and AA for the safest bonds and CCC for the riskier ones. But what happens in the case of bonds that have yet to be rated? How does one assess default risk in those cases? Proxying is the simple answer. Proxies are nothing more than crude guesses (sometimes plain guesses) of what the ratings should be. If this guess is too conservative, the bank is penalized through higher regulatory capital. But if it is too aggressive, regulators won’t approve the use of the proxies.
Enter data science. Given the availability of data on thousands of bonds rated over time by the rating agencies, we applied machine learning using a few attributes of those bonds (implied credit spread, time to maturity, etc.) in order to ‘train’ a predicative model. This training makes the model ‘learn’ how the agencies assign ratings to bonds. Subsequently, we used this model to ‘predict’ or infer what the ratings should be for the non-rated bonds (the test dataset).
The results could not have been better! We ran a backtesting exercise, where the model was applied back in time to non-rated bonds and had its results compared to ratings that were subsequently given by one of the rating agencies. The model achieved 87% accuracy on exact rating given by the agencies, and 98% on ratings within -1/+1 notches. These are extraordinary results by any standards in data science, not least because they were verified through backtesting rather than in-sample accuracy measures. And the good news does not stop there. We have successfully used data science for profiling term-deposit accounts, and are now exploring similar techniques for improving the infamous Value at Risk (VaR) measure of market risk on trading activities.
We are all riding through a one-way street when it comes to data use and applications in banking; there is no turning back. Those who resist will likely become less competitive and play a never-ending catch up game later on.
Regulatory capital on risk taking from bonds is calculated in line with the risk of the debtor to default on its obligations. This risk is normally assessed via the credit ratings assigned to those bonds by one of the three major rating agencies, Standard & Poors’, Moody’s and Fitch, comes in the form of categories such as AAA and AA for the safest bonds and CCC for the riskier ones. But what happens in the case of bonds that have yet to be rated? How does one assess default risk in those cases? Proxying is the simple answer. Proxies are nothing more than crude guesses (sometimes plain guesses) of what the ratings should be. If this guess is too conservative, the bank is penalized through higher regulatory capital. But if it is too aggressive, regulators won’t approve the use of the proxies.
Enter data science. Given the availability of data on thousands of bonds rated over time by the rating agencies, we applied machine learning using a few attributes of those bonds (implied credit spread, time to maturity, etc.) in order to ‘train’ a predicative model. This training makes the model ‘learn’ how the agencies assign ratings to bonds. Subsequently, we used this model to ‘predict’ or infer what the ratings should be for the non-rated bonds (the test dataset).
Given how critical some of banks’ internal technology systems are, there is also a natural lag in new IT infrastructure development
The results could not have been better! We ran a backtesting exercise, where the model was applied back in time to non-rated bonds and had its results compared to ratings that were subsequently given by one of the rating agencies. The model achieved 87% accuracy on exact rating given by the agencies, and 98% on ratings within -1/+1 notches. These are extraordinary results by any standards in data science, not least because they were verified through backtesting rather than in-sample accuracy measures. And the good news does not stop there. We have successfully used data science for profiling term-deposit accounts, and are now exploring similar techniques for improving the infamous Value at Risk (VaR) measure of market risk on trading activities.
We are all riding through a one-way street when it comes to data use and applications in banking; there is no turning back. Those who resist will likely become less competitive and play a never-ending catch up game later on.