
Learning Analytics & Educational Data Mining

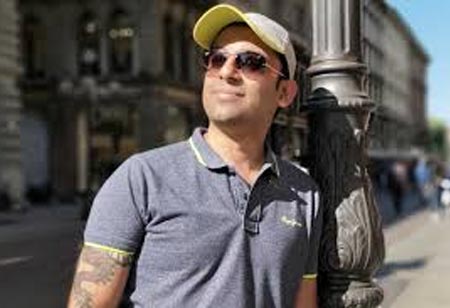
Jimit Dattani, CIO, XSEED Education, 0
Empirical evidence is required for theoretical frameworks to gain acceptance. During the past decade, the information overload ‘Big Data’ has led to the introduction and integration of new processing approaches. Data is the new gold and ensuring it is ‘unpolluted’ for analysis to derive insights is critical.
Why is it so important in educational reform?
Technology has revolutionized all aspects of our lives and modern education is becoming extensively reliant on technology for the development of critical, deep thinking skills and data insights. Learning Analytics (LA) has the power to enhance teaching and create more effective environments. Imagine you have all this data on how a student has performed over the course. Now, will it not be great if the data somehow could help to predict learners’ performance, provide insights that enabled the learning curve of teachers without whose support talking of education reform remains just so fragmentary. Empowering of teachers to make learning more effective is very core to educational reform.
We track student data in many ways from the digital trail they leave but the question is how can we tell from the digital profile if a student is learning? Other sectors are forging ahead using learning analytics and data mining, while the education sector is just get started. Adaptive and personalized learning becomes much more realistic when you’re able to derive so much from student data otherwise all that data is useless until institutions unlock its potential. To effectively harness the potential of learning analytics, data that often sits unused on servers and databases must be mined to a state where it speaks volumes. Learning analytics is gaining momentum and will continue to evolve and innovate quite rapidly, remember the whole point is from data mining to find positive actions that can be taken to spur improvement.
Learning Analytics (LA) focuses on applying tools and techniques at larger scales in instructional systems while Educational Data Mining (EDM) focuses on developing new tools and algorithms for discovering data patterns. If used properly, it can provide learners with a personalized eLearning experience, help schools increase retention rate, guide on how to improve the course structure and fuel the modern education system and pedagogy in particular.
How does all this work?
It all starts with the learning system where a student learns online and the system captures detailed data
about the student’s experiences. This Big Data then can be used to make predictions about future performance. Students can then receive personalized material to fuel the performance growth and teachers can intervene and help as necessary. As with most technological advancements, there are costs and challenges which can yield a high return on investments by developing a culture that uses data in making instructional decisions, involve IT departments in data collection roadmap, start with focused areas where data will help and build from there. It is equally important to communicate with parents on how the data is being collected and will be used. There are a wide variety of current methods popular within educational data mining. These methods fall into the following general categories: prediction, clustering, relationship mining, a discovery with models, and distillation of data for human judgment. The first three categories are largely acknowledged to be universal across types of data mining whilst the fourth and fifth categories achieve particular prominence within educational data mining.
What is the goal?
Predicting learner’s behavior by improving student models, improving knowledge domain structure models, studying the most effective pedagogical support for student learning and finally establishing empirical evidence. These can be achieved by adapting psychometrics, employing statistical techniques, mining log data, face-to-face contacts, studying the psychology of how humans learn and using Intelligent Tutoring System (ITS). When working towards these goals it is important to think about all the stakeholders and not just the students. Consider the educators, researchers/ developers, and institutions.
How to keep the data “unpolluted” for effective data mining?
Data management for analytics is not the same thing as data management for an enterprise data warehouse as it adds value along the way by completing summarisations and adding metadata to variables. The process to keep the data clean is to simplify access to traditional and emerging data (try to minimise data movement between data sources and focus on improving governances), strengthen the data scientists’ arsenal with advanced analytics techniques, scrub data to build quality into existing data capture processes, shape data using flexible manipulation techniques and finally share metadata across data management and analytics domains.
Conclusion
LA and EDM ride on high expectations even though they are relatively new fields of research and prone to several issues that need to be addressed. Big Data with technological progress represents an important paradigm shift and offers multiple opportunities. Despite the promise and high expectations, their application in educational environments is faced with barriers, such as lack of a data-driven culture and comprehensive easy-to-use integrated tools for LMS.
It is equally important to communicate with parents on how the data is being collected and will be used
What is the goal?
Predicting learner’s behavior by improving student models, improving knowledge domain structure models, studying the most effective pedagogical support for student learning and finally establishing empirical evidence. These can be achieved by adapting psychometrics, employing statistical techniques, mining log data, face-to-face contacts, studying the psychology of how humans learn and using Intelligent Tutoring System (ITS). When working towards these goals it is important to think about all the stakeholders and not just the students. Consider the educators, researchers/ developers, and institutions.
How to keep the data “unpolluted” for effective data mining?
Data management for analytics is not the same thing as data management for an enterprise data warehouse as it adds value along the way by completing summarisations and adding metadata to variables. The process to keep the data clean is to simplify access to traditional and emerging data (try to minimise data movement between data sources and focus on improving governances), strengthen the data scientists’ arsenal with advanced analytics techniques, scrub data to build quality into existing data capture processes, shape data using flexible manipulation techniques and finally share metadata across data management and analytics domains.
Conclusion
LA and EDM ride on high expectations even though they are relatively new fields of research and prone to several issues that need to be addressed. Big Data with technological progress represents an important paradigm shift and offers multiple opportunities. Despite the promise and high expectations, their application in educational environments is faced with barriers, such as lack of a data-driven culture and comprehensive easy-to-use integrated tools for LMS.