
Transforming Business Processes With Intelligent Analytics

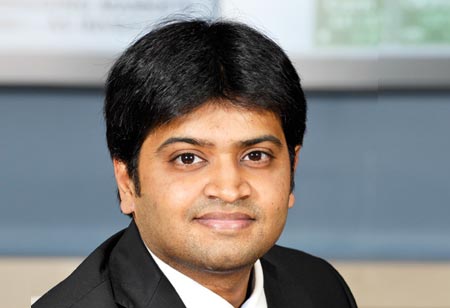
Srikanth Chakkilam, CEO, Cigniti Technologies, 0
Business transformation in the time of digital dominance is not only about changing your organizational culture, processes, tools, and technologies. Neither is it just about disrupting existing processes, nor does it entail only the formulation of new strategies. If you dig deeper to the core of it all, you will realize that this digital business transformation is actually about the shift from an intuitive way of decision-making to an informed one that makes businesses customer-centric and capable of delivering incredible customer experiences. The key factor that differentiates digital business transformation from the traditional business processes is the data-driven approach. With such a transformation, organizations aim to ensure availability of the required information and data anytime and anywhere.
Data has always been around us. There were multiple sources, reports, and surveys that offered ‘predictions’ for running a business. Now, when the volume of data has become surplus, intelligent analysis is performed on the massive datapool or big data to offer actionable insights, which empower businesses with the knowledge of accuracy and correctness of their decisions. These insights become the ultimate driver of the three phases of business transformation – Digital enablement, Digital optimization, and Digital transformation.
Certainly, the number of data sources has increased several folds with digitalization. The ability to sort and filter out the data, extract valuable information, and apply the derived insights flawlessly to the business processes, is what would segregate leaders from laggards in this digital age. Conventional database systems fall short when it comes to mining and analyzing the voluminous big data. They are designed to handle structured data but do not perform as well with semi-structured or unstructured data. Given the present market dynamics, we cannot disregard the unstructured data forms. As a matter of fact, the ability to mine unstructured and semi-structured data can offer the much-needed competitive edge to any business.
As data grows, the analytical capabilities should also increase for making the full use of the available data. Companies are using big data applications, driven by Artificial Intelligence and Machine Learning, for analyzing the data collected from various sources. These applications show the trends as well as the steps that the businesses should take to gain insights and make the right business decisions. However, this process of collect-analyze-act majorly relies on the validity and relevance of the sourced data.
Algorithms can run all the calculations on the information, you can have all sorts of bar graphs and trend mapping presented to you, but what if the data on which the calculations are performed is itself inaccurate? The failure rate of the big data projects is estimated to be an extremely concerning rate of around 85 percent. One cannot just take a heap of data, throw it up the wall
for mathematical scheming, and expect to turn the course of their business overnight.
The pragmatic way of implementing big data intelligence
Big data analytics, as opposed to the common belief, is no silver bullet. It is rather a meticulously-crafted, thoroughly-thought, and carefully-established business strategy, which is powered by the right infrastructure, the right leadership, and the right skills. In order to make sure that businesses get the best out of their investment in big data analytics, they need to:
• Create the right culture and have the right people onboard: Traditionally, executives have relied on their gut more than the available data to make decisions. This is one of the major reasons causing such a large number of failures of big data projects. The ‘right’ culture of big data involves a makeover of the organization in terms of the infrastructure, tools, human resources, and business approach. Before pouring out bags of gold to purchase machine learning and AI tools, it is necessary define clear business goals and then map the tools against them. The buying decision of a tool should be based on how well it aligns with your objectives – not the expensive features, not the sophisticated new updates – but the compatibility of your business processes and goals with the tool.
Once you finalize these changes, you need to make your people aware about them and then train them to leverage the opportunity to its fullest. Doing so will eradicate any resistance from your employees and will facilitate open embracing of the data-driven processes.
• Test, test, and test: Big data is characterized by gargantuan volume, unfathomable velocity, and extensive variety. The data is mostly unstructured, available in different formats of texts, pictures, videos, and more. Big data applications are capable of integrating information as and when it is generated. The predict capabilities of the big data applications can offer key business insights to speed ahead of the competition. But without AI and ML, big data is just big – heaps of information with no insights. Intelligent algorithms extract data from various sources, analyze it, and convert it into a legible format through classification, organization, and reasoning. For big data analytics to happen successfully, it is paramount to ascertain the reliability and cleanliness of data. This necessitates the employment of end-to-end testing of all the data sources as well as the integrators.
With employment of big data analytics into business processes, time is of essence, which is why instant data collection and deployment become highly critical. By testing the data applications and getting them certified for live deployment, businesses can stay assured of the viability of the obtained insights. Comprehensive and thorough testing, including data sampling and cataloging techniques, can help businesses achieve real-time scalability quickly.
When AI is integrated with the big data applications, the entire analytics process becomes faster, efficient, and accurate. The AI-driven big data analytics applications empower businesses to understand their customers better and make sense out of the available pools of information. Intelligent analytics not only transform business processes, but also bestows them with predict capabilities to scale ahead of the competition.
The pragmatic way of implementing big data intelligence
Big data analytics, as opposed to the common belief, is no silver bullet. It is rather a meticulously-crafted, thoroughly-thought, and carefully-established business strategy, which is powered by the right infrastructure, the right leadership, and the right skills. In order to make sure that businesses get the best out of their investment in big data analytics, they need to:
One cannot just take a heap of data, throw it up the wall for mathematical scheming, and expect to turn the course of their business overnight
• Create the right culture and have the right people onboard: Traditionally, executives have relied on their gut more than the available data to make decisions. This is one of the major reasons causing such a large number of failures of big data projects. The ‘right’ culture of big data involves a makeover of the organization in terms of the infrastructure, tools, human resources, and business approach. Before pouring out bags of gold to purchase machine learning and AI tools, it is necessary define clear business goals and then map the tools against them. The buying decision of a tool should be based on how well it aligns with your objectives – not the expensive features, not the sophisticated new updates – but the compatibility of your business processes and goals with the tool.
Once you finalize these changes, you need to make your people aware about them and then train them to leverage the opportunity to its fullest. Doing so will eradicate any resistance from your employees and will facilitate open embracing of the data-driven processes.
• Test, test, and test: Big data is characterized by gargantuan volume, unfathomable velocity, and extensive variety. The data is mostly unstructured, available in different formats of texts, pictures, videos, and more. Big data applications are capable of integrating information as and when it is generated. The predict capabilities of the big data applications can offer key business insights to speed ahead of the competition. But without AI and ML, big data is just big – heaps of information with no insights. Intelligent algorithms extract data from various sources, analyze it, and convert it into a legible format through classification, organization, and reasoning. For big data analytics to happen successfully, it is paramount to ascertain the reliability and cleanliness of data. This necessitates the employment of end-to-end testing of all the data sources as well as the integrators.
With employment of big data analytics into business processes, time is of essence, which is why instant data collection and deployment become highly critical. By testing the data applications and getting them certified for live deployment, businesses can stay assured of the viability of the obtained insights. Comprehensive and thorough testing, including data sampling and cataloging techniques, can help businesses achieve real-time scalability quickly.
When AI is integrated with the big data applications, the entire analytics process becomes faster, efficient, and accurate. The AI-driven big data analytics applications empower businesses to understand their customers better and make sense out of the available pools of information. Intelligent analytics not only transform business processes, but also bestows them with predict capabilities to scale ahead of the competition.