
Solving Data Science Problems from First Principles

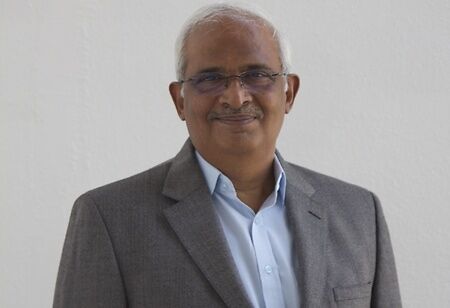
Dr. T. Ravindra Babu, Global Head, Data Science, Sahaj Ai, 0
With massive amounts of data being collected at every second in our daily life, the importance for building models over such data to provide business advantage, as well as, to provide improved personal comforts is increasing. Some data collection scenarios include that when we visit an online store to make a purchase, those systems capture the time that we spend on the home page, the items that we view, the time we take to make a decision to purchase or not making a purchase, time we spend on the content shown by the company, etc. Some other data collection touch points are online content viewing, the daily route that we travel, songs that we listen to, etc. Such data is in turn used to create better customer experience for the customers by showing relevant advertisements, and personalized recommendations, while at the same time providing commercial value to those who serve.
Vague Problem Statements
On most occasions, especially, in the startup world, the problem statements emanating from business teams remain vague, when posed to data scientists. Some examples are “the customer addresses are noisy, can you do something about it?”, “the users are not getting converted (not making purchases online) and how could data science models help”, “the catalog content gets manually curated, can machine learning algorithms automate this”, “who should we extend the option to buy through installments”, and “how can we detect and solve online fraud”. At this stage, it is left to the maturity of data scientists to convert them into a formal mathematical statement, seek data that captures these signals, and build models.
Solving from First Principles
A data scientist approaches to solve the above problems in multiple ways, such as, whether such a problem was solved earlier in research literatures, solutions in other industrial settings as well as from the first principles. On most occasions, the problems are unique and need specific attention as well as solutions. Also, it is practically found that one could only adapt abstract ideas from such literature rather than replicating them. The first principles approach relates to understanding the problem on the ground and its domain; and finding a solution through formal machine learning principles.
Let us discuss one problem of customer addressing challenges. With a broad direction provided by the vague business problem, a data scientist needs to understand actual challenges on the ground, get a hands-on feel about the data, decide on what kind of model could be built to solve the problem, and how it could be deployed and measured through the entire modeling life cycle. This needs visits to mother hubs and delivery hubs to understand how shipments are received, distributed among the delivery agents before delivering to the customers. Discussion with delivery agents and managers provides insights to the challenges. At this stage,in the parlance of machine learning, a decision is made on supervised or unsupervised learning approaches to be chosen to solve these problems, and challenges that need to be surmounted for their deployment in hubs. On most occasions one chooses to solve using supervised learning algorithms since they could be measured effectively. A model is built on these considerations and deployed.
Summary
Data Science model building requires domain understanding, obtaining data insights and finding a solution that is apt for the given scenario from the first principles. First principles approaches are most effective. While developing the solution it is necessary to visualize the final operational scenario, latency requirements and challenges in the adaptation by the relevant stakeholders.
A data scientist approaches to solve the above problems in multiple ways, such as, whether such a problem was solved earlier in research literatures, solutions in other industrial settings as well as from the first principles. On most occasions, the problems are unique and need specific attention as well as solutions. Also, it is practically found that one could only adapt abstract ideas from such literature rather than replicating them. The first principles approach relates to understanding the problem on the ground and its domain; and finding a solution through formal machine learning principles.
While developing the solution it is necessary to visualize the final operational scenario, latency requirements and challenges in the adaptation by the relevant stakeholders
Let us discuss one problem of customer addressing challenges. With a broad direction provided by the vague business problem, a data scientist needs to understand actual challenges on the ground, get a hands-on feel about the data, decide on what kind of model could be built to solve the problem, and how it could be deployed and measured through the entire modeling life cycle. This needs visits to mother hubs and delivery hubs to understand how shipments are received, distributed among the delivery agents before delivering to the customers. Discussion with delivery agents and managers provides insights to the challenges. At this stage,in the parlance of machine learning, a decision is made on supervised or unsupervised learning approaches to be chosen to solve these problems, and challenges that need to be surmounted for their deployment in hubs. On most occasions one chooses to solve using supervised learning algorithms since they could be measured effectively. A model is built on these considerations and deployed.
Summary
Data Science model building requires domain understanding, obtaining data insights and finding a solution that is apt for the given scenario from the first principles. First principles approaches are most effective. While developing the solution it is necessary to visualize the final operational scenario, latency requirements and challenges in the adaptation by the relevant stakeholders.