
An Expert's Guide to Data Annotation in AI/ML Environments

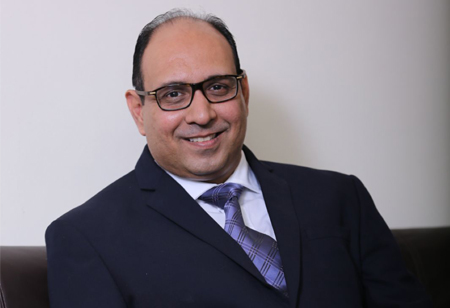
Neville Patel, CEO, Qualitas Global, 0
Briefly explain the importance of data annotation for the growth of IT companies dealing with AI/ML.
Data annotation is crucial for all companies delving in Machine Learning and AI. Annotating or Labeling raw datasets forms the basis for machine model development and refinement, fostering the creation of diverse and representative datasets necessary for handling complex tasks. High-quality annotations ensure the accuracy and reliability of machine learning models, directly impacting their effectiveness.
What are some of the key advantages that data annotation offers for various AI/ML models?
Data annotation significantly boosts AI and ML model performance in several key ways. Firstly, it provides ground truth labels for supervised learning algorithms, enhancing accuracy and reliability for real-world applications. Additionally, it brings-forth diverse datasets, mitigates biases and improves model adaptability across different scenarios. Furthermore, it enables specialized machine model development tailored to specific domains, leading to targeted solutions. Thus, it is safe to say that data annotation is foundational for building robust AI and ML models that will empower innovation across various industries.
Throw some light on the common challenges that AI/ML companies face in terms of data annotation.
While these companies face a myriad of complex challenges in their data annotation process, the most common, yet significant ones are in terms of scalability, quality assurance, domain expertise and cost-effectiveness in their data annotation processes. While maintaining high-quality annotations is essential to
prevent errors that can affect machine model performance, scaling annotation processes for large datasets while ensuring consistency and accuracy is an uphill task for most enterprises. Also, domain expertise is critical for accurate annotation of specialized industry-wide datasets. However, manual annotation can be costly and time-consuming.
Tell us about the primary characteristics that data annotation specialists must imbibe to develop solutions that cater to diverse requirements.
Data annotation specialists are vital for scaling AI capabilities and meeting market demands. However, staying updated on emerging AI/ML trends is crucial for these specialists to be able to employ the latest tools and methodologies. Also, they should prioritize efficient annotation workflows for increasing throughput. Additionally, having domain-specific expertise ensures the quality and relevance of annotations, which can be achieved through collaboration with stakeholders and domain experts. Furthermore, continuous improvement and quality assurance practices, including rigorous validation and user feedback, enhances dataset reliability and machine model performance.
How do you expect data annotation to shape-up in the years to come?
Going forward, data annotation is expected to further accelerate AI/ML solutions development across diverse verticals driven majorly by increased automation, efficiency, and scalability. One key trend will be the automation of annotation processes using AI-powered tools and algorithms, which employ techniques like computer vision and deep learning to speed-up annotation and reduce manual intervention. Also, active learning techniques will optimize annotation efficiency by having models select the most informative data points. Furthermore, advancements in crowd annotation platforms and distributed computing will enable quick and cost-effective annotation of large datasets. Additionally, semi-supervised and self-supervised learning approaches are promising for learning from partially annotated or unlabeled data. Overall, the future of data annotation will undergo significant transformation due to technological advancements.
Tell us about the primary characteristics that data annotation specialists must imbibe to develop solutions that cater to diverse requirements.
Data annotation specialists are vital for scaling AI capabilities and meeting market demands. However, staying updated on emerging AI/ML trends is crucial for these specialists to be able to employ the latest tools and methodologies. Also, they should prioritize efficient annotation workflows for increasing throughput. Additionally, having domain-specific expertise ensures the quality and relevance of annotations, which can be achieved through collaboration with stakeholders and domain experts. Furthermore, continuous improvement and quality assurance practices, including rigorous validation and user feedback, enhances dataset reliability and machine model performance.
Data will continue to be the key to higher productivity and continued growth in the business environments. Just as humans upskill to thrive, so too will AI need to keep learning from data gathered and labelled accurately.
How do you expect data annotation to shape-up in the years to come?
Going forward, data annotation is expected to further accelerate AI/ML solutions development across diverse verticals driven majorly by increased automation, efficiency, and scalability. One key trend will be the automation of annotation processes using AI-powered tools and algorithms, which employ techniques like computer vision and deep learning to speed-up annotation and reduce manual intervention. Also, active learning techniques will optimize annotation efficiency by having models select the most informative data points. Furthermore, advancements in crowd annotation platforms and distributed computing will enable quick and cost-effective annotation of large datasets. Additionally, semi-supervised and self-supervised learning approaches are promising for learning from partially annotated or unlabeled data. Overall, the future of data annotation will undergo significant transformation due to technological advancements.